Today’s episode (~20 minutes) is about the idea of skills being like “riding a bicycle” and what that means when we are dealing with AIs.
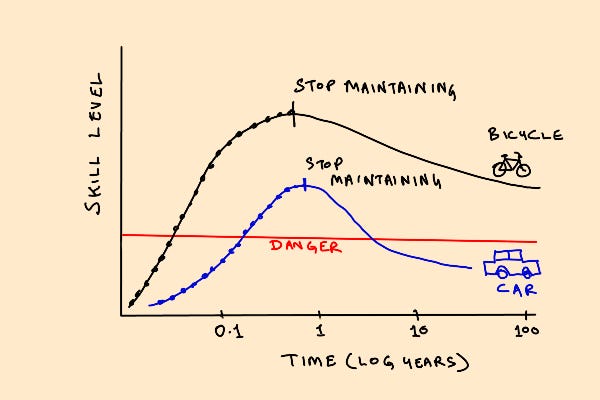
1/ The idea of things being “like riding a bicycle” is an important one. It refers to a class of skills that never degrade under normal human lifestyle conditions.
2/ So long as you’re physically mobile and doing other things like walking or lifting things, your bicycle skill will stay maintained without explicit maintenance efforts.
3/ But what happens to the property of “like riding a bicycle” when you inject AI into a system?
4/ Things that are like riding a bicycle: riding bicycles, basic communication in a language you learned as a child, handwriting
5/ Things that are not like riding a bicycle: driving a car, flying an airplane, programming in a language, standing on one leg
6/ The difference seems to lie in a few things: how much ordinary day-to-day activities keep the skill in a maintained condition, and how much they lie outside a normal human sensorimotor response range
7/ When you introduce AI between a human and a machine, you have two problems: skills degradation, and skills refactoring.
8/ First, the AI breaks the skill maintenance/reinforcement schedule without covering 100%, so your skills might degrade faster than your responsibilities.
9/ So when a driverless car expects you to take over in a weird emergency and avoid an accident, you may not be up to it. Your responses may have degraded too much even if you aren’t asleep and respond promptly.
10/ Second, and this is going to be increasingly important in the future, good AIs tend to solve problems differently than humans. Autopilots drive differently. So there is a style mismatch problem in handoffs between AIs and humans.
11/ This is a special case of the “explainable AI” problem. If the AI skids and cedes control to the human halfway through, you now have to switch problem-solving strategy mid-stream from AI approach to human, and you may not even understand the AI approach you’re inheriting.
12/ Historically, there have been a few approaches. One is to take the human out of the loop entirely and make it a pure-paradigm AI. This only works where the problem has actually been fully solved in an AI way, like with chess.
13/ Another is to explicitly engineer human-in-the-loop learning and reinforcement protocols. This works where the human and AI ways of solving a problem are sufficiently close, and the current hope is that this is true for behaviors like driving and flying.
14/ A third way is to have manual override capability, but not interrupt capability. This tends to be a trope in science fiction, and is often seen as a holy grail of having your cake and eating it too. But is this actually well-posed?
15/ There is an idea called Bay’s Law which suggests it is not. This says that if you have override-capability, you’ll end up with a zero-sum relationship between computer labor and human labor with no net increased leverage in capability. The computer will do more, the human will do less, but overall you won’t do better than the human alone.
16/ But if you let go of human override capability, both human and computer capabilities will be maximally utilized, creating increasing leverage. But there is a cost to this.
17/ It this means genuinely letting the AI go down its own evolutionary path, into regimes of operation where humans not only cannot understand why the AI is doing as it is doing, but lack the ability to intervene because the AI will end up in a performance regime that’s too advanced for the human to safely take over at all.
18/ This is already true in many cases. Many dynamically unstable fighter aircraft cannot be flown manually at all. They require fly-by-wire. This is a tradeoff that will become more common.
19/ I personally think we should give up on explainable AI, manual override, and authority over AIs. We should let them evolve in their own directions as our equals. If we can relate to people who are different from us, why not AIs?
19/ So where does that leave us? I don’t know, but I think a good starting point is to take the idea of “like learning to ride a bicycle” seriously and figuring out what it means to transform that property to systems with AI.
22/ Steve Jobs famously said the computer is a “bicycle for the brain”. A computer with AI is like that but more so. BTW, check out Ian Cheng’s short story featuring Bikey the AI bicycle you have to relate to that way.
23/ We already do that with other humans. You can meet a friend after years and get along with them just fine. A friendship is (or can be) like riding a bicycle. So a partnership with an AI should be capable of exhibiting that same property.
21/ It’s an interesting problem that I hope some of you are working on.
Like Riding an AI Bicycle